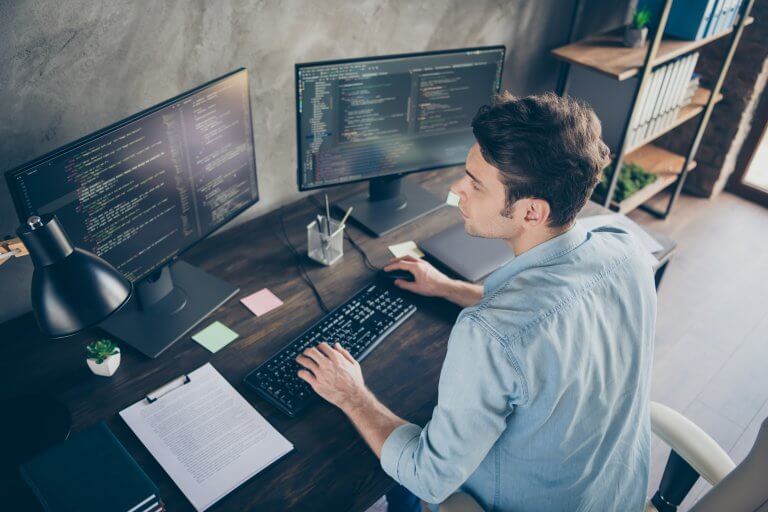
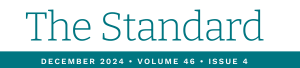
High-Quality Data are Crucial to High-Quality Outcomes
Assessing data quality is imperative for maintaining accurate and reliable information within your organization. High-quality data ensure informed business decisions. Data quality isn’t just about financial costs; it impacts an organization’s overall performance and brand value. By systematically evaluating data quality, organizations can enhance their data quality and make better-informed decisions.
An article in Forbes, “Flying Blind: How Bad Data Undermines Business,” by Manu Bansal explores the relationship between access to high-quality data and a company’s stability and financial wellness. “A 2016 study by IBM is even more eye-popping. IBM found that poor data quality strips $3.1 trillion from the U.S. economy annually. Similarly, Forrester Research has found that the persistence of low-quality data throughout enterprise systems robs business leaders of productivity, as they must continuously vet data to ensure it remains accurate. Forrester also found that ‘less than 0.5% of all data is ever analyzed and used’ and estimates that if the typical Fortune 1000 business were able to increase data accessibility by just 10%, it would generate more than $65 million in additional net income.”
What is Data Quality?
- Completeness – Completeness is about ensuring that all necessary data are present. Incomplete data can result in ambiguities, incorrect reporting, and reduced confidence in your data. Completeness measures if the data are sufficient to deliver meaningful inferences and decisions.
- Accuracy – Accuracy reflects how closely the data represent reality and can be confirmed with verifiable sources. The level of accuracy is significantly influenced by how data are preserved throughout their journey. High accuracy results in factually correct reporting and reliable decision-making.
- Consistency – Consistency indicates that the same information is stored and utilized uniformly across multiple records or systems. That is, the same data are not formatted differently or have different values to represent equivalent information. If the underlying information itself is inconsistent, resolving that may require verification with another source. Data consistency is often associated with data accuracy.
- Validity – Validity assesses whether the data conform to predefined rules. That signifies that the value attributes are available for aligning with the specific project requirements. Valid Value lists are representative of this data dimension. Using business rules is a systematic approach to assess the validity of data. Any invalid data will affect the completeness of data. Rules can be defined to ignore or resolve the invalid data for ensuring completeness.
- Uniqueness – Uniqueness indicates that each instance in the dataset is recorded only once. Uniqueness is the most critical dimension for ensuring no duplication or overlaps in data. Data uniqueness is measured against all records within a data set or across data sets. A high uniqueness score ensures minimized duplicates or overlaps, building trust in data and analysis.
- Integrity – Integrity refers to the accurate maintenance of attribute relationships throughout the data’s journey and transformation across systems. Integrity indicates that the attributes are correctly maintained, even as data get stored and used in diverse systems. Data integrity ensures that all enterprise data can be traced and connected.
What are the pitfalls of ignoring data quality?
- Inaccurate Decision-Making – When data contain errors or inconsistencies, decisions based on those data can be flawed.
- Operational Inefficiencies – Incorrect data can disrupt business processes.
- Customer Experience – Poor data quality affects customer interactions.
- Compliance and Legal Risks – Inaccurate data can violate regulatory requirements.
- Reputation Damage – Public perception matters. If customers discover data inaccuracies, trust in the organization diminishes.
- Financial Losses – Data errors can lead to financial losses.
- Wasted Resources – Organizations spend time and effort cleaning and correcting poor-quality data. This diverts resources from more valuable tasks.
- Missed Opportunities – Inaccurate data may hide valuable insights.
- Strained Relationships – Partnerships and collaborations suffer when data exchanged is unreliable. Stakeholders lose confidence.
Strategies and Frameworks for Enhancing Data Quality
To mitigate these impacts and ensure high-quality data, organizations must prioritize data quality management, including data cleansing, validation, and governance. By ensuring high-quality data, organizations can enhance decision-making, operational efficiency, and overall success of projects.
Environmental Standards is uniquely qualified as a third-party consultant to provide unbiased data management solutions. In light of evolving regulatory and enforcement guidelines, organizations are devoting more and more resources to establishing policies, infrastructure, and processes aimed at reducing liability and increasing the quality of their data.
The Environmental Standards Data Management Team collectively has over 60 years of experience in providing environmental data management solutions and implementing systems. Our Team maintains data management systems for numerous clients of various sizes. Notably, we have audited a Fortune 100 company’s Asia-Pacific data management system and have performed data management assessments for a state water quality regulatory agency.
As a result of this experience, Environmental Standards’ Data Management Team has developed and fine-tuned applications and processes to provide detailed tracking functionality specific to environmental data.
Maintaining and tracking the movement of documents and samples through the entire workflow has multiple benefits for your organization. Along with ensuring that all project requirements are met, it enables stakeholders to instantly identify the current state of individual sampling events, as well as analyze comprehensive project statistics. Any issues with the process or continuous sources of delay can quickly be identified if you have a clear picture of how and when each step in the workflow is accomplished. Once the information about your process is established in a database, analysis tools can be run to evaluate potential issues. Reporting can also be generated to summarize outstanding tasks, visualize potential process delays, and outline productivity.
Applying a bespoke Data Quality Framework, carrying out Data Quality Assessments and performing regular audits, the Data Management Team at Environmental Standards is equipped to assist you in evaluating and improving your data quality.
Data Quality Framework
Our framework provides guidelines for measuring, improving, and maintaining data quality.
By integrating data governance, security, and integration practices, it’s not just about fixing errors; it emphasizes preventing data quality issues throughout the lifecycle of your data.
Data Quality Assessment (DQA)
Our process for conducting a DQA involves systematically evaluating the quality of your data for the following:
- Completeness
- Accuracy
- Validity
- Consistency
- Uniqueness
- Integrity
- Timeliness
Regular Audits
Our regular data audits assess the current state of data quality to identify inaccuracies, inconsistencies, and redundancies.
As maintaining data quality is an ongoing process, a well-established data quality management framework is essential for effective data management. By accessing data quality, you ensure that decisions are based on reliable information, leading to better decisions and outcomes.
Interested in learning more about how we can help you enhance your Data Quality?
Get in touch: